Rameshbabu Manyam
Research Assistant Professor
Research Assistant Professor
Faculty, Biostatistics and Bioinformatics
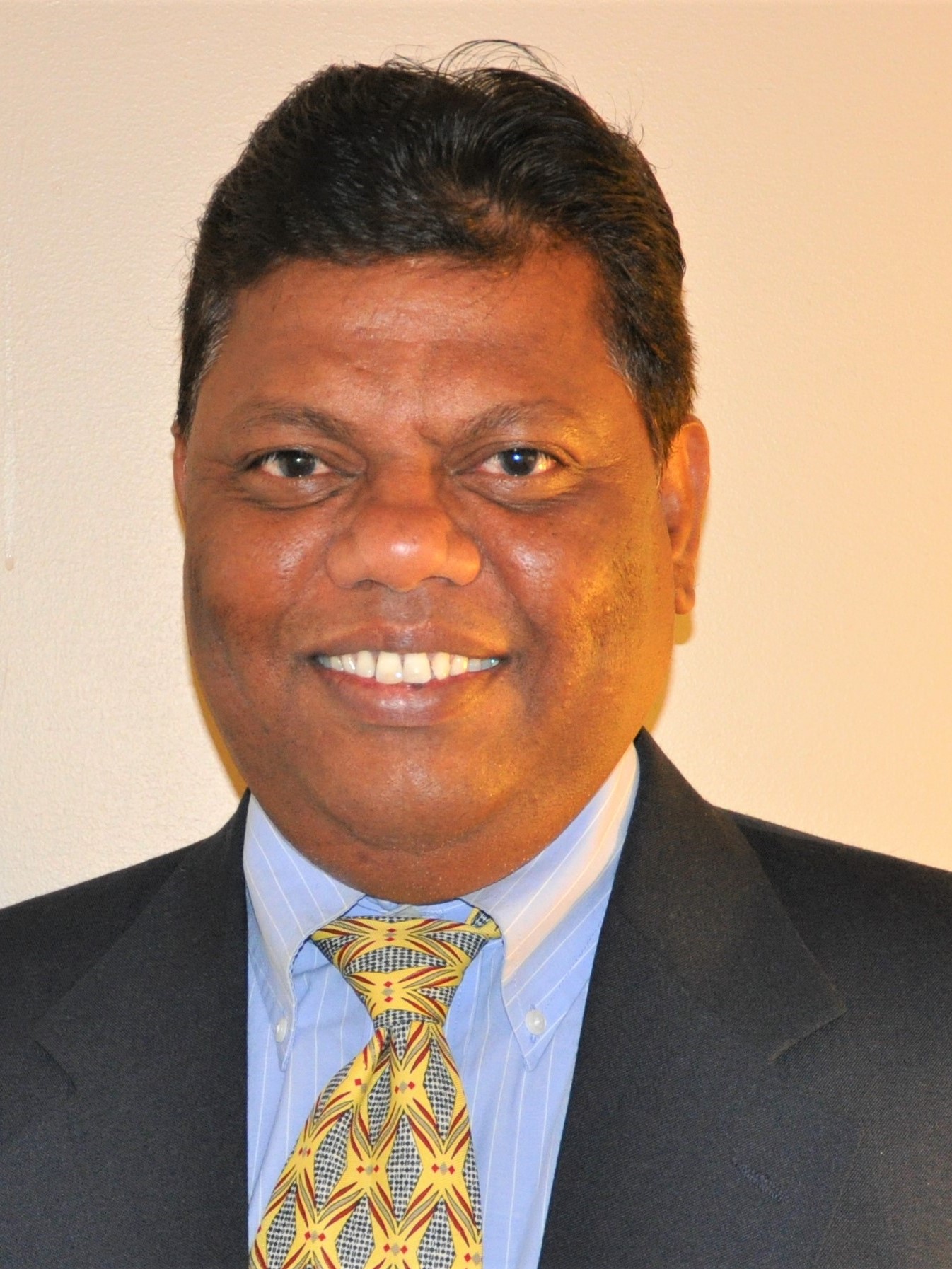
Dr. Manyam's research focuses on data science, database management, big data analytics, and risk prediction frameworks using machine learning algorithms and high-performance computing environments. Specific fields of interest include longitudinal EMR data analytics using statistical and machine learning and AI algorithms (e.g., survival regression. decision trees, eXtreme Gradient Boosting: XGBoost, random survival forests, support vector machines, and neural networks).
Dr. Manyam has over 25 years of professional experience working in several data-driven research projects and building custom databases, software applications, and data visualization portals. He has hands-on working knowledge with Emory healthcare data sources (such as Cerner’s Electronic Medical Records (EMR), EPIC’s Electronic Health Records (EHR) database systems, the society of thoracic surgeons’ institutional adult cardiac surgical database and clinical data warehouse).
Dr. Manyam's current collaborative research efforts include, but not limited to: 1) developing scalable, portable, and reproducible machine learning powered frameworks - via feature engineering, hybrid feature selection, survival analysis and cutting-edge ensemble learning techniques - to accurately predict risk factors for (a) Failure-to-Rescue after coronary artery bypass grafting (CABG), (b) hospital readmission after CABG, (c) mortality after surgery for femoral shaft fractures, and d) blood transfusion for patients who undergo surgery for femoral shaft fractures; 2) developing personalized HIV testing frequency recommendations based on AI-powered recommendation engine, 3) analyzing EHR data to evaluate differences in the utilization of outpatient rehabilitation services among young adults with stroke (YAS) between the ages 21-60 years old, and 4) analyzing Medicare claims data from the Centers for Medicare and Medicaid Services (CMS) to develop machine learning-powered risk models for a) primary care fragmentation, b) interhospital transfer and c) early mortality after interhospital transfer in older adults.
Recent honors and awards
- 2024: 1st place Mike Kutner Faculty Poster Award Winner and Travel Award Recipient at the Southern Regional Council on Statistics (SRCOS) Summer Research Conference
- 2023: Dean's Pilot Innovation Award, Emory Rollins School of Public Health
- 2022: Biostatistics and Bioinformatics Faculty Mentor Award, Emory University
- 2021: Biostatistics and Bioinformatics Distinguished Teaching Award, Emory University
- 2020: Outstanding Graduate Student Award, Dept.of Computer Science, Georgia State Univeristy
- 2019: Three Minute Thesis (3MT) event second place winner, Georgia State University
Contact Information
Department of Biostatistics and Bioinformatics, Rollins School of Public Health, Emory University
Atlanta , GA 30322
Email: rmanyam@emory.edu
Areas of Interest
- Analytics
- Artificial Intelligence
- Bioinformatics
- Cardiovascular Diseases
- Data Mining
- Data Science
- High Performance Computing
- Longitudinal Analysis
- Machine Learning
- Modeling
- Risk Assessment
- Survival Analysis
Education
- PhD 2019, Georgia State University, Atlanta, GA, USA
- MS 2002, Georgia State University, Atlanta, GA, USA
- MTech 1993, Indian Institute of Technology, Banaras Hindu University, Varanasi, India
- BTech 1991, National Institute of Technology, Warangal, India
Affiliations & Activities
- 2024-25: Faculty Advisor for Emory Humphrey Fellow
- 2024: Georgia Statistics Day, Emory University
- 2024: IEEE International Conference on Bioinformatics and Biomedicine
- 2023: Mentor for PhD Consortium at Georgia Statistics Day, Georgia Institute of Technology
- Spring 2023: Python workshop in collaboration with the student organization, Rollins mHealth Collaborative (RmC)
- Fall 2022 : SQL workshop in collaboration with RmC