Return to Faculty Listing
David Benkeser
Associate Professor
Faculty, Biostatistics and Bioinformatics
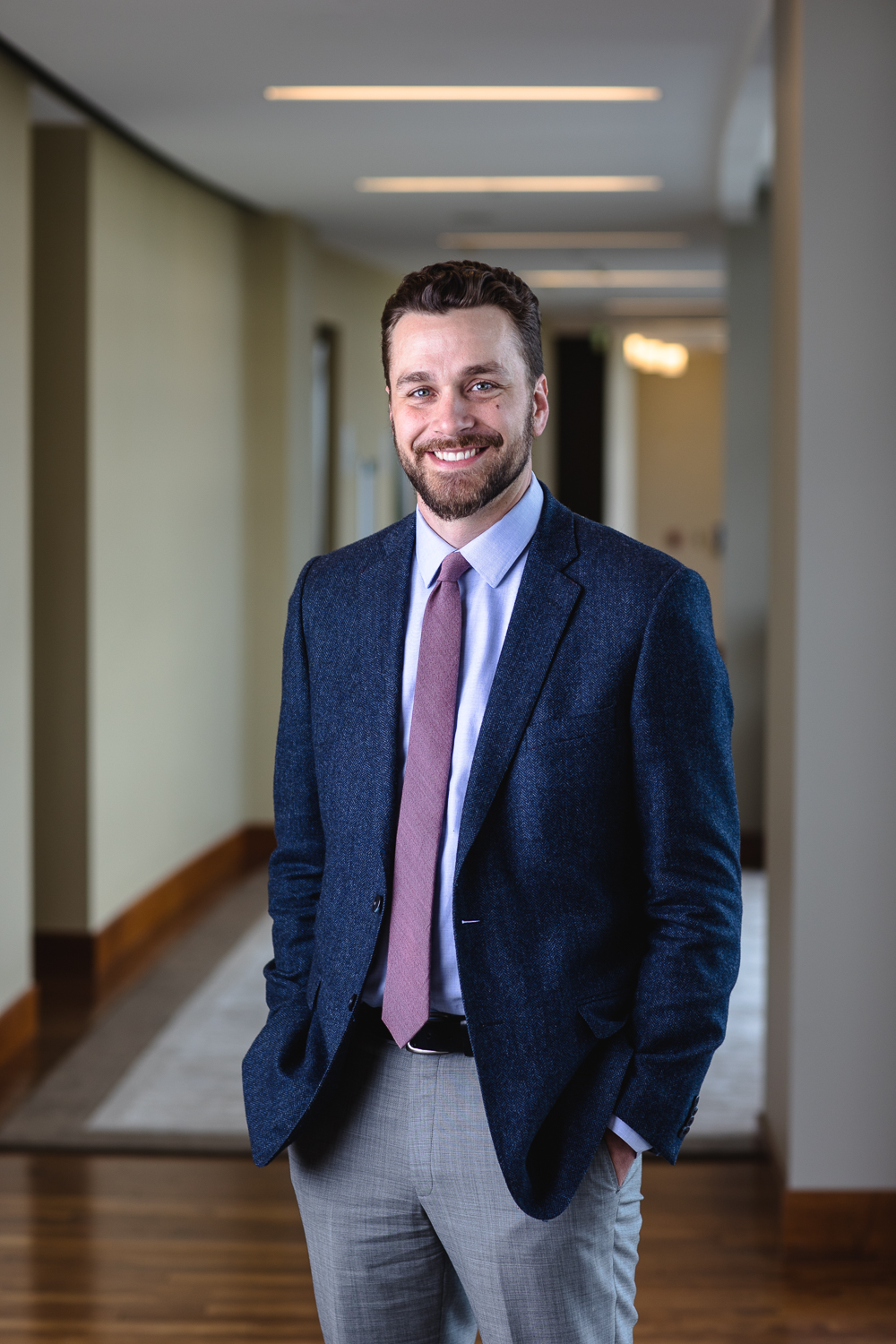
My methodological research focuses on the theory and applications of machine learning in causal inference. Specific areas of interest include vaccine statistics, competing risks, complex longitudinal data, and theory of robust nonparametric statistical inference. My collaborative research includes work on preventive vaccines, tuberculosis, and HIV prevention.
Contact Information
1518 Clifton Road, N.E.
Atlanta , GA 30322
1518-002-3AA
Email: benkeser@emory.edu
Areas of Interest
- Causal Inference
- HIV/AIDS Prevention
- Infectious Disease
- Machine Learning
- Survival Analysis
- Vaccines
Education
- B.S. 2010, University of Georgia
- MPH 2010, University of Georgia
- PhD. 2015, University of Washington
Courses Taught
- DATA 550: Data Science Toolkit
- DATA 555: Current Topics in Data Science